Understanding AI Transparency for Better Trust and Accountability
Understanding AI transparency has quickly become one of the most important topics in today’s tech-driven world. As artificial intelligence continues to influence every sector, the demand for transparency in AI is crucial for fairness, ethical decision-making, and public trust.
AI transparency refers to the clarity around how AI operates, how decisions are made, and how data is handled. This article covers key elements that help build trust and accountability in AI, including the benefits, challenges, and strategies to make AI more transparent.
1. What is AI Transparency?
At its core, AI transparency is about being clear and open about how artificial intelligence models work. Unlike traditional software, which follows a set of explicit rules, AI models are often “black boxes” due to their complexity. Transparency in AI can be divided into three main types:
- Process Transparency: Understanding how AI is developed, including model selection, training data, and intended use.
- Outcome Transparency: Clarity on how AI arrives at its decisions or predictions.
- Data Transparency: Disclosure of the types of data used to train the model, including data sources and privacy measures taken.
This combination of transparency ensures that stakeholders understand how AI systems function, providing a basis for trust and confidence.
2. Importance of Transparency in Building Trust
Building trust is vital for the widespread adoption of AI technologies. Users need assurance that AI systems make ethical and unbiased decisions.
Transparency helps establish this trust by making AI systems’ operations understandable to all stakeholders.
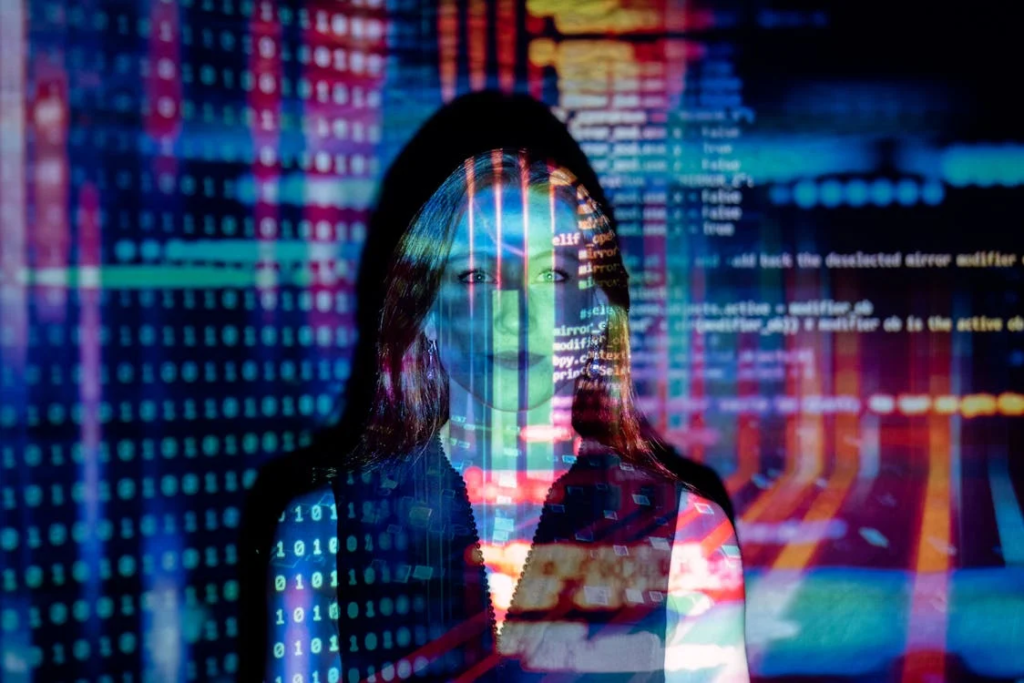
For example, when AI systems provide clear explanations, it boosts users’ confidence and promotes a sense of security.
In sectors such as healthcare, increased AI transparency has shown significant improvements in patient trust and engagement.
3. What AI Experts Say About Accountability, Trust and Transparency
Scientists like Timnit Gebru and Yoshua Bengio emphasize the importance of accountability and trust in AI because these elements are critical to ensuring that AI systems are fair, ethical, and beneficial for society.
Timnit Gebru advocates for greater transparency in AI to ensure that models are not only understandable but also inclusive of diverse perspectives, which helps prevent biases and promotes fairness.
Yoshua Bengio, a pioneer in AI research, stresses that transparency in AI decision-making fosters trust, enabling users to better understand how AI systems work and hold them accountable.
Both experts recognize that without accountability and transparency, AI could perpetuate harm, create inequalities, or undermine public confidence, making it essential for AI development to be both open and responsible.
Here’s a list of other AI experts advising about understanding AI transparency.
Expert | Quote | Expertise |
---|---|---|
Stuart Russell | “If we want AI to be beneficial, we must design it to be transparent, understandable, and controllable.” | AI researcher, professor at UC Berkeley, expert in AI safety and ethics |
Yoshua Bengio | “To build trust in AI, we need transparency in how decisions are made and ensure that algorithms are accountable.” | AI pioneer, professor at the University of Montreal, co-recipient of the Turing Award |
Timnit Gebru | “For AI to be trustworthy, we must not only make the models transparent, but also include diverse voices in the process.” | AI researcher, advocate for diversity in AI, co-founder of Black in AI |
Kate Crawford | “Transparency in AI isn’t just about revealing the inner workings of a model; it’s about ensuring that its impacts are visible and accountable to society.” | Senior principal researcher at Microsoft Research, AI ethics expert |
Judea Pearl | “Trustworthy AI requires not only transparency in decision-making but also the ability for humans to understand and intervene when necessary.” | Computer scientist, philosopher, expert in causal reasoning and AI theory |
4. AI Accountability
In the context of AI, accountability means taking responsibility for the outcomes AI produces, whether positive or negative.
Understanding AI transparency supports accountability in areas like data privacy, bias, and fairness.
For instance, when AI models are transparent, it’s easier to trace and address any biases in their decisions.
Legal guidelines, such as the General Data Protection Regulation (GDPR) and the upcoming EU AI Act, emphasize the need for responsible and accountable AI, requiring developers to disclose how their models work and what data they use.
Image Source : commission.europa.eu
5. Benefits of AI Transparency
AI transparency provides multiple benefits across various sectors:
- Increased User Confidence: When users understand how AI operates, they’re more likely to trust and rely on these systems.
- Enhanced Decision Quality: Knowing an AI system’s limitations allows users to make better-informed decisions.
- Reduced Bias and Improved Fairness: Transparency helps expose biases and enables developers to reduce or eliminate them.
- Promotes Ethical AI Development: With clear standards and ethics in place, transparency motivates developers to uphold high ethical standards.
6. Challenges in Achieving AI Transparency
Despite its importance, achieving AI transparency is not without challenges:
- Technical Complexity: AI models are often complex, making them hard to explain in simple terms.
- Intellectual Property Concerns: Companies may be reluctant to share proprietary model details, fearing loss of competitive advantage.
- Data Privacy: Disclosing data used in AI models raises privacy concerns, especially in sensitive areas like healthcare or finance.
- Interpretability vs. Accuracy: Simplifying models to improve transparency can sometimes reduce their effectiveness, posing a dilemma between interpretability and performance.
7. Strategies to Increase Trust in AI
There are several strategies that organizations can implement to improve AI transparency:
Developing Explainable AI (XAI): This approach focuses on creating AI models that provide clear, human-understandable explanations for their predictions. Explainable AI is especially critical in sensitive fields like healthcare and law, where understanding the basis for AI decisions is essential.
Use of Transparency Reports: Providing regular transparency reports on AI models allows users to understand how these models function, how they are monitored for fairness, and how they align with ethical standards. These reports help build user trust by making the processes more visible.
Open-Source Initiatives: Making AI algorithms and models open-source enables external experts to review, test, and assess them. This openness not only boosts accountability but also fosters community-driven improvements, enhancing model reliability and reducing potential biases.
Engagement with Stakeholders: Involving diverse stakeholders, such as users, regulators, and ethical boards, in the development and deployment of AI ensures that the systems meet public expectations for transparency and fairness. Stakeholder input is invaluable for making AI systems more inclusive and aligned with societal values.
Implementing Model Documentation: Creating detailed documentation for each AI model—including its purpose, limitations, data sources, and potential biases—enables both developers and users to better understand the model’s design and intended application. This documentation serves as a resource for transparency and accountability.
Using Interactive Explanations: Interactive interfaces that allow users to explore how AI reached specific decisions, using tools like visual breakdowns and simulations, help make complex AI models more accessible and understandable. These explanations build user confidence by clarifying the decision-making process.
Adopting Bias Audits and Fairness Checks: Conducting regular audits and fairness evaluations helps identify and mitigate biases within AI systems. Sharing audit results with the public demonstrates a commitment to ethical standards and fairness, enhancing the organization’s accountability and credibility.
Establishing an AI Ethics Board: Forming an ethics board within organizations to oversee AI development ensures adherence to ethical standards and transparency throughout the design and deployment process. These boards bring in diverse perspectives and offer recommendations on best practices for ethical and accountable AI use.
8. Case Studies: AI Accountability in Action
Several industries have seen transparency improve trust and accountability in AI:
- Healthcare: Transparent AI systems in medical diagnostics have helped patients understand treatment options, improving trust in AI-driven recommendations.
- Tech Company Backlash: A lack of transparency in data usage led to major public outcry against certain tech companies. This case highlights the importance of AI transparency for reputation.
- Finance: Financial institutions that prioritize AI transparency have gained user trust, as transparency in models predicting creditworthiness and loan eligibility ensures fairness.
9. The Future of AI Transparency
AI transparency is moving toward becoming a standard practice, with increased calls for ethical and accountable AI. As regulations tighten, developers are likely to integrate transparency into the core design of AI models. Future developments in transparency could significantly impact AI adoption in areas like government, finance, and healthcare, where trust is critical.
FAQs
1. What is AI transparency and explainability?
AI transparency refers to the degree to which the decision-making process of an AI system can be understood and traced by humans. Explainability, a key aspect of transparency, means the ability to clearly communicate how an AI model makes its predictions or decisions in a way that is understandable to non-experts. Both concepts are crucial for building trust, ensuring accountability, and improving the fairness of AI systems.
2. Can you provide some examples of AI transparency in action?
Examples of AI transparency include companies like IBM and Google making their AI models more accessible through explainable AI (XAI) initiatives. For instance, IBM’s Watson Health uses AI transparency to show how decisions in healthcare are made, while Google’s AI models offer insights into how they arrive at specific outcomes. Another example is the use of transparency reports by organizations to regularly update stakeholders on how AI systems operate and are monitored.
3. What is an AI transparency statement?
An AI transparency statement is a formal declaration by an organization detailing how its AI systems work, including the data used, the decision-making processes, and the potential impacts on users. It is designed to provide users and stakeholders with insight into the ethical considerations, limitations, and fairness of the AI systems deployed, ensuring that AI development is conducted responsibly.
4. What are the consequences of the lack of transparency in artificial intelligence?
The lack of transparency in AI can lead to various issues, such as biased decisions, unfair practices, and erosion of public trust. When AI systems operate as “black boxes” without clear explanations, it becomes difficult to identify and address errors or biases. This can result in discriminatory outcomes, legal and ethical concerns, and a general reluctance to adopt AI technologies.
5. What is an AI transparency framework?
An AI transparency framework is a structured set of guidelines or principles aimed at ensuring AI systems are transparent and explainable. These frameworks typically include principles such as providing clear documentation, making decision-making processes auditable, and ensuring user access to understand the models. The framework helps organizations build AI systems that are more accountable, ethical, and aligned with public interest.
6. How does understanding transparency in AI ethics benefit society?
Transparency in AI ethics ensures that AI technologies are developed and deployed in ways that are just, fair, and aligned with societal values. By making AI systems transparent, stakeholders can assess their ethical implications, such as privacy concerns and bias in decision-making. This openness encourages responsible innovation, ensures accountability, and fosters trust, helping AI to benefit society as a whole while minimizing harm.
Related Posts
Cultivating Trust in The AI Search Age: The Power of Authenticity
AI-Generated Content: Trustworthy Innovation or Digital Deception?
Maintaining Authenticity in the Age of AI: Strategies for Bloggers
Dodging AI’s Jab: How the Impact of AI is Hitting the Workforce
Conclusion
Understanding AI transparency is essential to building trust and ensuring accountability in modern AI systems.
The combination of transparency, explainability, and stakeholder engagement is key to a future where AI operates ethically and fairly.
Developers, regulators, and users must collaborate to support transparent AI, as this will play a pivotal role in society’s broader adoption of and confidence in artificial intelligence.