Fair AI Content for Trust: A Guide to Ethical AI Systems
Fair AI content for trust is becoming a non-negotiable demand in today’s tech-driven world. Did you know that nearly 80% of AI models show some form of bias?
This startling fact underscores the importance of developing AI systems that are not only accurate but also fair and transparent.
As AI becomes a bigger part of our everyday lives—shaping decisions on hiring, lending, and healthcare—ensuring its fairness is crucial for fostering trust and inclusivity.
In this article, we’ll break down how to create fair AI content that builds trust, addresses key challenges, and provides actionable solutions.
Whether you’re a developer, business leader, or AI enthusiast, you’ll walk away with insights to ensure your AI systems are ethical, transparent, and truly inclusive.
Keep reading to discover the practical steps you can take today to lead the charge for fair AI.
What is Fairness in AI?
Fairness in AI means designing systems that make decisions impartially, equitably, and justly. Think of it like a referee in a game—neutral, consistent, and free from favoritism.
For example, in healthcare, AI can help prioritize patient care. But what happens if the AI model is trained on data that underrepresents minorities?
It may unintentionally prioritize some groups over others.
Similarly, in hiring, AI systems may prefer male candidates if trained on biased historical data.
This is where fairness comes into play, ensuring systems operate without prejudice.
Is Fairness a Goal of AI?
Yes, fairness is at the heart of ethical AI practices.
Many AI initiatives focus on building systems that promote transparency and trust.
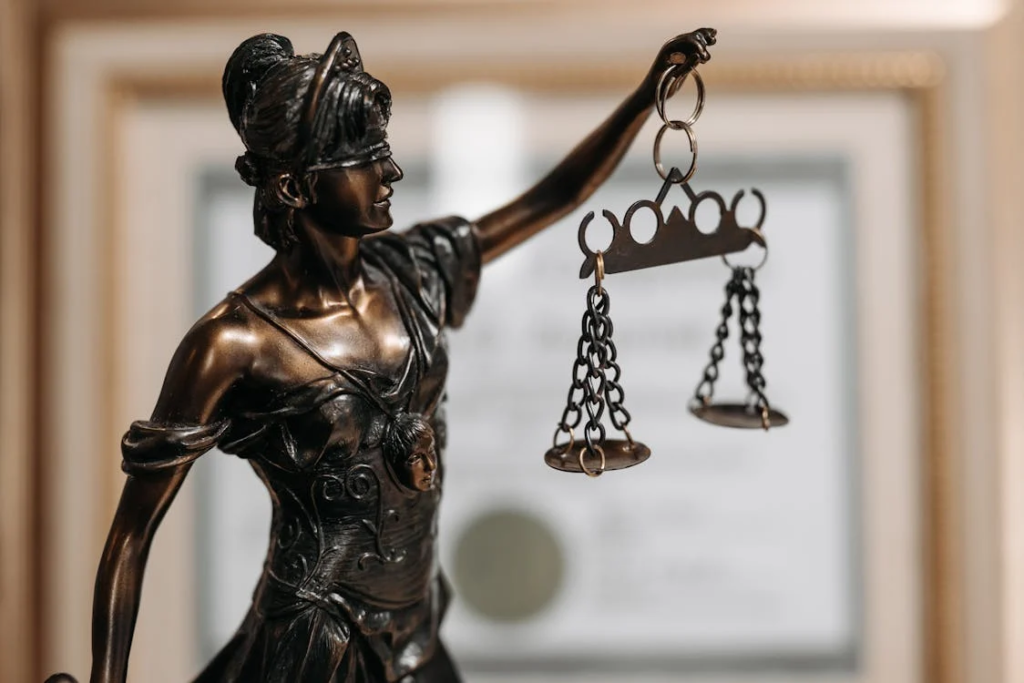
For example, voluntary fairness assessments are now a standard practice, where developers evaluate AI models for potential biases before they’re deployed.
Fairness is not just a nice-to-have—it’s a critical pillar of ethical AI. Without it, AI risks perpetuating inequality and reinforcing existing biases.
By ensuring fairness, AI can be a powerful tool for empowering individuals and creating a more just and inclusive society.
Why Fairness Matters in AI
The Growing Influence of AI
Imagine a world where AI decides who gets a job, an apartment, or even justice. Sounds futuristic, right? The truth is, it’s already happening.
AI is becoming increasingly influential in decision-making across various industries.
Fairness Drives Public Trust
Fairness isn’t just about being ethical; it’s about building trust with the public.
If people perceive AI as biased, it undermines their confidence in its ability to make fair decisions.
When trust is lost, AI’s intended purpose is compromised.
Creating Equitable Systems
Fairness in AI means creating systems that treat all individuals equally, regardless of their gender, ethnicity, or socio-economic background.
Without fairness, AI could become a gatekeeper, reinforcing biases it was meant to eliminate, instead of leveling the playing field.
Ethical Imperative for Fairness
Fairness in AI is not a luxury; it’s essential. Every person deserves equal moral consideration.
AI should be a tool for uplifting humanity, not reinforcing inequality or discrimination.
The Dangers of Bias in AI Decisions
Bias in AI can lead to harmful and discriminatory outcomes.
For instance, a recruiting tool that consistently overlooks women for leadership roles perpetuates gender inequality.
Such biases deepen societal divisions and hinder progress toward equality.
Eroding Public Confidence
Discriminatory outcomes from AI not only perpetuate injustice but also erode public trust.
People need to see AI working impartially to believe in its value.
Without fairness, AI risks losing its credibility and becoming a source of division rather than progress.
The Principle of Fairness in AI
Fairness starts with a simple idea: AI systems must process data in ways people would expect. In short, to build trust, AI should be explainable, and AI should also be transparent.
It’s like playing a game where everyone knows the rules upfront.
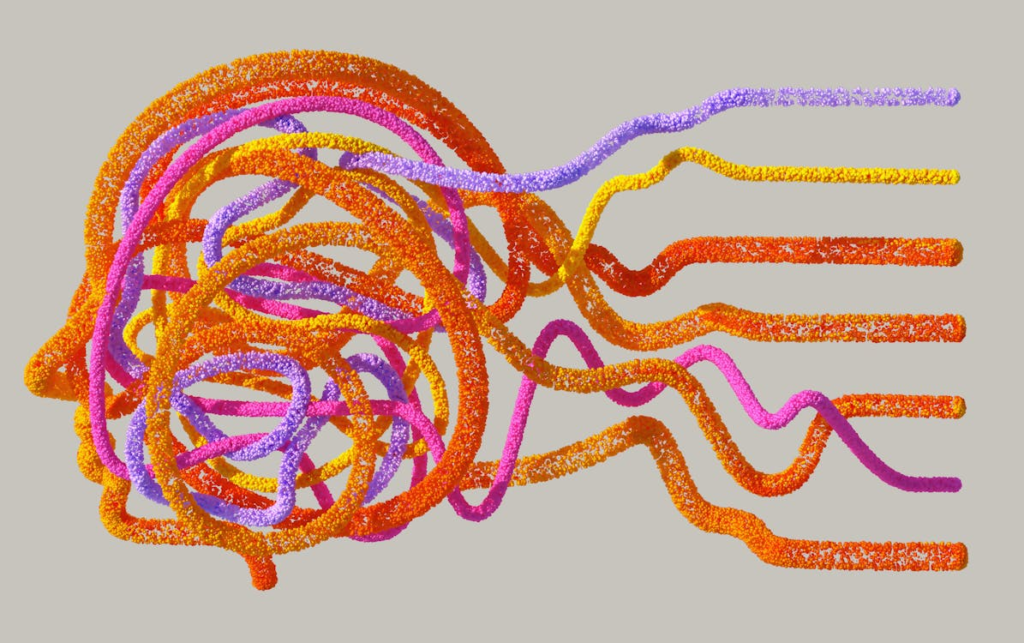
However, fairness goes beyond expectations. It ensures AI systems avoid unjustified adverse effects.
If an algorithm determines loan eligibility, fairness means it shouldn’t deny loans based on a person’s zip code or ethnicity.
Transparency and accountability are critical to achieving this principle.
How to Make Fair AI Content?
Creating fair AI content is like building a house—it requires a strong foundation, careful planning, and collaboration. Here’s how we can make it happen step by step:
1. Start with Diverse and Inclusive Datasets
AI systems learn from the data they’re fed. If this data only represents certain groups, the AI will likely produce biased outcomes.
To create fair AI content, it’s essential to use datasets that reflect the world’s diversity.
This means including information from people of various genders, ethnicities, and socio-economic backgrounds.
Think of it like cooking a balanced meal—you need ingredients from all food groups to ensure everyone is nourished.
2. Ensure Transparent Algorithms
Imagine trying to trust a magician who never reveals their tricks.
Similarly, AI systems need to be transparent about how they work.
Developers must document and explain the processes behind their algorithms.
By doing so, they create accountability, much like a recipe that lists every step and ingredient.
Transparency ensures that users and regulators can understand and trust the decisions AI makes.
3. Incorporate Ethical Design Principles
Fair AI content should be designed with ethical principles at its core.
This involves prioritizing inclusivity, fairness, and user-centric outcomes during the development phase.
Ethical design is like drawing a blueprint that ensures safety and comfort for everyone using the space.
It’s not just about functionality—it’s about creating a system that respects and benefits all users equally.
4. Test Regularly for Bias
AI systems are not a “set it and forget it” technology. Continuous testing is crucial to identify and mitigate any biases that may emerge.
Developers should perform fairness audits at different stages of the content lifecycle. It’s like checking the quality of a product before shipping it—ensuring it meets the standards and expectations of fairness.
5. Promote Global Collaboration
Fairness in AI is not limited to one country or region.
It’s a global challenge that requires international cooperation.
By sharing knowledge, resources, and best practices, countries can create universally fair AI standards.
This collaboration is like a symphony—each instrument plays its part to create harmony across borders.
6. Leverage Feedback from Diverse Users
Feedback is invaluable when it comes to improving AI fairness.
Engaging users from diverse backgrounds helps identify potential blind spots in AI content.
This process is like having multiple pairs of eyes review a manuscript to catch errors that one person might miss.
Listening to users ensures that the AI meets their needs without unintentionally excluding or disadvantaging anyone.
7. Educate and Empower Stakeholders
Lastly, fairness in AI content requires informed stakeholders.
Developers, businesses, and users need education about the ethical implications of AI.
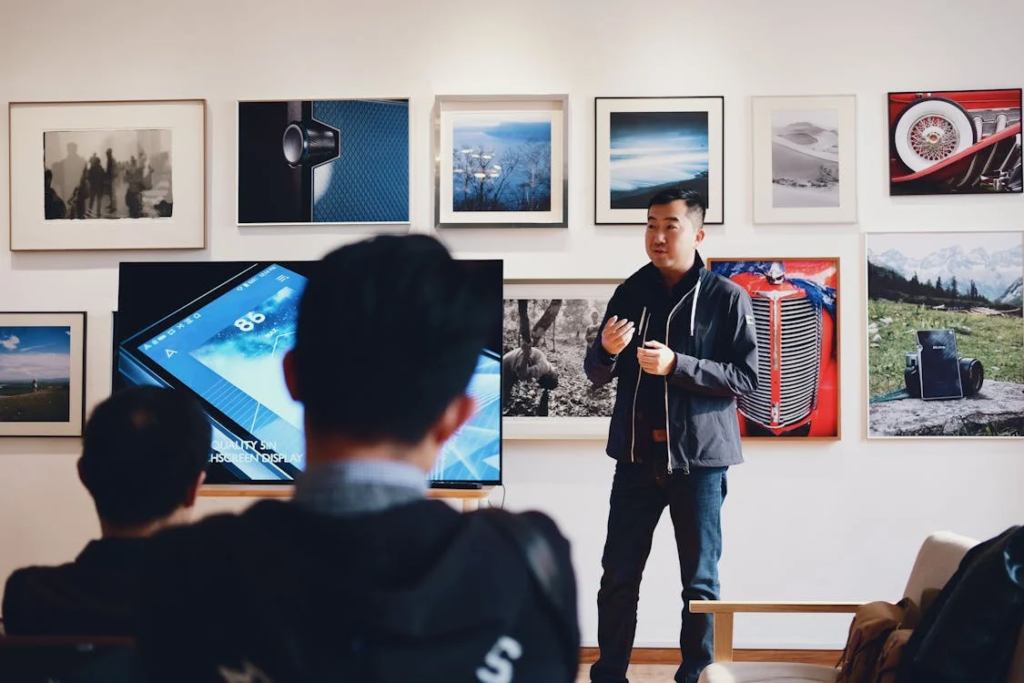
Workshops, guidelines, and training programs can empower them to make better decisions.
Think of this step as teaching someone to fish instead of giving them fish—it creates a self-sustaining system where everyone plays a role in promoting fairness.
Key Focus Area: Fair AI Content
Key Focus Area | Description | Analogy |
---|---|---|
Diverse and Inclusive Datasets | Use data from various genders, ethnicities, and socio-economic groups to avoid bias. | Like using all colors to paint a complete picture. |
Transparent Algorithms | Ensure algorithms are documented and decisions are explainable to build accountability. | Like following a step-by-step recipe. |
Ethical Design Principles | Embed inclusivity and fairness into the development phase of AI systems. | Like creating a blueprint for safety and comfort. |
Regular Bias Testing | Continuously audit AI for fairness throughout its lifecycle to identify and fix biases. | Like checking a product for quality before releasing it. |
Global Collaboration | Encourage countries to share knowledge and establish universal ethical AI standards. | Like a symphony where every instrument plays a role in harmony. |
User Feedback Integration | Involve diverse users to catch blind spots and ensure inclusive AI content. | Like multiple eyes proofreading a manuscript for errors. |
Stakeholder Education | Train developers, businesses, and users on ethical AI practices to empower fair decision-making. | Like teaching someone to fish for self-reliance. |
Challenges to Achieving Fairness in AI
1. Bias in Datasets
Historical data often reflects human biases, which AI systems inadvertently learn and perpetuate.
For instance, underrepresentation of women in tech hiring datasets leads to unfair outcomes in recruitment decisions.
2. Algorithmic Biases
AI models can amplify existing prejudices in training data, worsening inequalities.
Facial recognition software, for example, often struggles with darker skin tones, leading to inaccurate and biased results.
3. Lack of Transparency
Many AI models function as “black boxes,” making their decision-making processes difficult to interpret.
This lack of clarity leaves users questioning decisions, such as why a loan application was denied.
4. Limited Accountability
Few regulations enforce responsibility for unfair outcomes in AI systems.
Companies often evade consequences for biased algorithms, further weakening public trust in AI technologies.
5. Global Disparities
Developing countries frequently lack the resources and infrastructure to build fair AI systems.
This creates a gap in access to ethical AI, deepening inequalities between nations.
By addressing these challenges systematically, we can pave the way for AI systems that are equitable and trustworthy for everyone.
Creating fair AI is easier said than done.
How to Measure Fairness in AI
Measuring fairness in AI is a complex yet critical task.
It’s like solving a puzzle, where each piece contributes to the bigger picture.
Let’s explore some practical ways to assess fairness and ensure ethical AI development.
1. Data Collection
The foundation of fair AI lies in the quality and diversity of its data.
Datasets must represent a wide range of demographics, including genders, ethnicities, and socioeconomic backgrounds.
For example, if an AI model for hiring decisions lacks representation of women or minority groups, it could lead to biased outcomes.
2. Feature Selection
Carefully selecting input variables is key to minimizing bias.
Developers should exclude features that may indirectly reflect protected attributes, such as race or gender.
For instance, using ZIP codes in loan approvals can unintentionally reflect racial or economic disparities.
3. Model Training
Fairness must be monitored throughout the training process.
Developers should test algorithms for bias during development and recheck them after deployment.
Regular audits can identify and fix problems before they affect real-world decisions.
4. Bias Detection Tools
Several tools are available to measure and mitigate bias in AI systems.
IBM’s AI Fairness 360 and Google’s What-If Tool enable developers to evaluate their models’ fairness and make necessary adjustments.
These tools are invaluable in maintaining transparency and accountability.
5. Performance Audits
Once the model is deployed, continuous monitoring is essential.
Fairness can evolve as new data is introduced, so regular audits ensure the system remains unbiased. It’s like maintaining a car—routine checks prevent long-term issues.
6. User Feedback
Finally, fairness can also be measured through user experience.
Listening to feedback from diverse users provides valuable insights into how the AI performs across different demographics, ensuring inclusivity and trust.
Measuring Fairness in AI Content
Step | Description | Example |
---|---|---|
Data Collection | Ensure datasets are diverse and representative. | Include data from various genders and ethnic groups. |
Feature Selection | Avoid using variables that perpetuate biases. | Exclude ZIP codes in loan approval models. |
Model Training | Test for bias during and after development. | Identify and address bias in hiring algorithms. |
Bias Detection Tools | Use tools to assess and adjust fairness. | Utilize IBM’s AI Fairness 360 for regular audits. |
Performance Audits | Monitor models continuously to maintain fairness. | Conduct quarterly fairness reviews for AI decision-making. |
User Feedback | Incorporate insights from diverse users to improve models. | Analyze feedback from users in underrepresented communities. |
Fairness as an Ethical and Balanced Outcome
Imagine a judge who makes decisions solely based on facts, unaffected by personal biases. Fair AI systems should aim to replicate this impartiality. Safeguards against favoritism ensure decisions are ethical, balanced, and inclusive.
This is particularly important in sensitive domains like:
- Recruitment: Ensuring diverse hiring practices.
- Lending: Avoiding biases in credit approvals.
- Education: Providing equal access to learning opportunities.
Building Trust Through Fair AI Practices
1. Fairness Drives Trust
When AI systems treat everyone equally, they earn trust from users. This trust is essential for wide adoption and ensures that technology benefits all users fairly.
2. A Level Playing Field
Fair AI eliminates biases, creating a level playing field for all individuals. This equal treatment fosters inclusivity and reduces disparities, promoting social equity.
3. AI as a Reflection of Values
Fair AI is not just about algorithms—it reflects society’s collective values. By designing systems that are unbiased, we ensure that AI aligns with our shared ideals of justice and fairness.
4. Transparency and Accountability
Transparent AI practices help users understand how decisions are made. When people can trust that AI decisions are explainable and fair, they are more likely to use and embrace the technology.
5. Building a Fairer Future
The goal of fair AI is to create a future where everyone has equal access and opportunities. By embedding fairness in AI systems, we pave the way for more ethical, equitable, and inclusive societies.
FAQs
- What is fair AI content for trust?
Fair AI content ensures unbiased decisions that build user confidence and trust. - Why is fairness in AI important?
Fairness prevents discrimination, treats everyone equally, and fosters trust in AI systems. - How can AI developers ensure fairness?
By using diverse datasets, transparent algorithms, and regularly testing for biases. - What challenges affect fairness in AI?
Bias in datasets, algorithmic prejudice, and lack of transparency are major hurdles. - How do you measure AI fairness?
Through methods like fairness-aware data collection, feature selection, and model evaluations. Such measurements help create fair AI content and trust. - Why do AI systems become biased?
Bias often stems from skewed or incomplete training data reflecting historical inequalities. - What is the role of government in AI fairness?
Governments can enforce ethical standards, conduct fairness assessments, and promote inclusive AI practices.
Related Posts
Preserving Human Control in AI-Assisted Decision-Making
Ethical AI Practices Made Easy: 7 Simple Steps for Success
Understanding AI Transparency for Better Trust and Accountability
How AI Content Earns Our Trust One Step at a Time
Conclusion
Fair AI content for building trust isn’t a destination; it’s a journey.
To build trust, developers, governments, and users must work together. Transparency, diversity, and accountability are the cornerstones of fair AI practices.
The future of AI holds incredible promise. But for it to empower everyone equally, fairness must remain at the heart of its development.
Let’s aim for an AI-powered world that uplifts, inspires, and treats all individuals with dignity.